AI Assurance: Towards Trustworthy, Explainable, Safe, and Ethical AI
- 9h 38m
- Feras A. Batarseh, Laura Freeman
- Elsevier Science and Technology Books, Inc.
- 2022
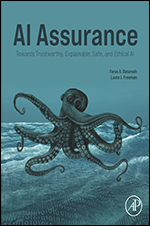
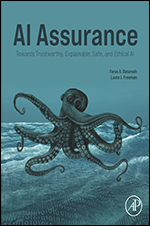
AI Assurance: Towards Trustworthy, Explainable, Safe, and Ethical AI provides readers with solutions and a foundational understanding of the methods that can be applied to test AI systems and provide assurance. Anyone developing software systems with intelligence, building learning algorithms, or deploying AI to a domain-specific problem (such as allocating cyber breaches, analyzing causation at a smart farm, reducing readmissions at a hospital, ensuring soldiers’ safety in the battlefield, or predicting exports of one country to another) will benefit from the methods presented in this book.
As AI assurance is now a major piece in AI and engineering research, this book will serve as a guide for researchers, scientists and students in their studies and experimentation. Moreover, as AI is being increasingly discussed and utilized at government and policymaking venues, the assurance of AI systems—as presented in this book—is at the nexus of such debates.
- Provides readers with an in-depth understanding of how to develop and apply Artificial Intelligence in a valid, explainable, fair and ethical manner
- Includes various AI methods, including Deep Learning, Machine Learning, Reinforcement Learning, Computer Vision, Agent-Based Systems, Natural Language Processing, Text Mining, Predictive Analytics, Prescriptive Analytics, Knowledge-Based Systems, and Evolutionary Algorithms
- Presents techniques for efficient and secure development of intelligent systems in a variety of domains, such as healthcare, cybersecurity, government, energy, education, and more
- Covers complete example datasets that are associated with the methods and algorithms developed in the book
About the Author
Feras A. Batarseh is an Associate Professor with the Department of Biological Systems Engineering at Virginia Tech (VT) and the Director of A3 (AI Assurance and Applications) Lab. His research spans the areas of AI Assurance, Cyberbiosecurity, AI for Agriculture and Water, and Data-Driven Public Policy. His work has been published at various prestigious journals and international conferences. Additionally, Dr. Batarseh published multiple chapters and books, his two recent books are: "Federal Data Science", and "Data Democracy", both by Elsevier’s Academic Press. Dr. Batarseh is a senior member of the Institute of Electrical and Electronics Engineers (IEEE), the Agricultural and Applied Economical Association (AAEA), and the Association for the Advancement of Artificial Intelligence (AAAI). He has taught AI and Data Science courses at multiple universities including George Mason University (GMU), University of Maryland - Baltimore County (UMBC), Georgetown University, and George Washington University (GWU). Dr. Batarseh obtained his Ph.D. and M.Sc. in Computer Engineering from the University of Central Florida (UCF) (2007, 2011), a Juris Masters of Law from GMU (2022), and a Graduate Certificate in Project Leadership from Cornell University (2016). He currently holds courtesy appointments with the Center for Advanced Innovation in Agriculture (CAIA), National Security Institute (NSI), and the Department of Electrical and Computer Engineering at VT.
Dr. Laura Freeman is a Research Associate Professor at the Department of Statistics and the Director of the Intelligent Systems Lab at Virginia Tech’s Hume Center. Her research leverages experimental methods for conducting research that brings together cyber-physical systems, Data Science, Artificial Intelligence, and Machine Learning to address critical challenges in national security. She is a CCI fellow.
In this Book
-
An Introduction to AI Assurance
-
Setting the Goals for Ethical, Unbiased, and Fair AI
-
An Overview of Explainable and Interpretable AI
-
Bias, Fairness, and Assurance in AI—Overview and Synthesis
-
An Evaluation of the Potential Global Impacts of AI Assurance
-
The Role of Inference in AI—Start S.M.A.L.L. with Mindful Modeling
-
Outlier Detection Using AI—A Survey
-
AI Assurance Using Causal Inference—Application to Public Policy
-
Data Collection, Wrangling, and Pre-Processing for AI Assurance
-
Coordination-Aware Assurance for End-to-End Machine Learning Systems—the R3E Approach
-
Assuring AI Methods for Economic Policymaking
-
Panopticon Implications of Ethical AI—Equity, Disparity, and Inequality in Healthcare
-
Recent Advances in Uncertainty Quantification Methods for Engineering Problems
-
Socially Responsible AI Assurance in Precision Agriculture for Farmers and Policymakers
-
The Application of Artificial Intelligence Assurance in Precision Farming and Agricultural Economics
-
Bringing Dark Data to Light with AI for Evidence-Based Policymaking